         
Tutorial Sessions/Invited Talks
All tutorials and invited talks are free to registered
conference attendees of all conferences held at
WOLDCOMP'12. Those who are interested in attending one
or more of the tutorials are to sign up on site at the
conference registration desk in Las Vegas. A complete &
current list of WORLDCOMP Tutorials
can be found
here.
In addition to tutorials at other conferences,
DMIN'12 aims at providing a set of tutorials dedicated
to Data Mining topics. The 2007 key tutorial was given
by Prof. Eamonn Keogh on Time Series Clustering. The
2008 key tutorial was presented by Mikhail Golovnya
(Senior Scientist, Salford Systems, USA) on Advanced
Data Mining Methodologies. DMIN'09 provided four
tutorials presented by Prof. Nitesh V. Chawla on Data
Mining with Sensitivity to Rare Events and Class
Imbalance, Prof. Asim Roy on Autonomous Machine Learning,
Dan Steinberg (CEO of Salford Systems) on Advanced Data
Mining Methodologies, and Peter Geczy on Emerging
Human-Web Interaction Research. DMIN'10 hosted a
tutorial presented by Prof. Vladimir Cherkassky on
Advanced Methodologies for Learning with Sparse Data. He
was a keynote speaker as well (Predictive Data Modeling
and the Nature of Scientific Discovery). In addition, we
had one tutorial held by Peter Geczy on Web Mining.
DMIN'12 will be hosting the following
invited talks:
Invited Talks
Invited Talk A |
Speaker |
Sofus A.
Macskassy, Univ. of Southern California, USA |
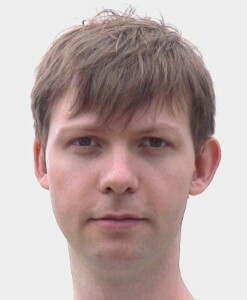 |
Topic/Title |
Mining Social
Media: The Importance of Combining Network and
Content |
Date & Time |
Monday, July 16,
03:20pm (45 min talk and Q&A) |
Location |
Ballroom 1 |
Description |
Use of Social
Media platforms is growing at an alarming rate.
Over the past years, we have seen a continuous
growth in the amount of social media data being
generated as well as increasing sophistication
and complexity of social media platforms. This
has spawned a vibrant community, both in
academia and industry, looking to make sense of
what is going on. While the amount of attention
in this space is growing, the analytic
techniques are still in their infancy and the
research questions are still quite narrow. In
fact, most work is focused either on the social
networks or on the content and the individual
users. I will in this talk try to expand beyond
the majority of current research and discuss how
we can make use of both the content and the
network to better understand and mine social
media. Specifically, I will here talk about how
we can automatically generate psychographic
profiles of users based on analyzing their
generated content, and then how we can use these
models to better explain their observed
retweeting behaviors. |
Short Bio |
Sofus A. Macskassy
is a Sr. Computer Scientist at the Information
Sciences Institute and an Adjunct Professor of
Computer Science at the University of Southern
California. He was previously the Director of
Fetch Labs at Fetch Technologies. His work spans
a wide area of research and domains, including
social media analytics, where he is extracting
and analyzing user profiles and behaviors from
Twitter. His main research areas include
statistical relational learning, information
filtering, data mining and social network
analysis. He is published in top venues in AI,
ML and DM and has served on numerous organizing
and programming committees. He serves on the
editorial board of the Machine Learning Journal,
the premier machine learning journal in the
world. He is also the primary developer and
maintainer of the open-source Network Learning
Toolkit for Statistical Relational Learning. |
Invited Talk B |
Speaker |
Haym Hirsh,
Rutgers University, USA |
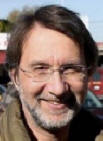 |
Topic/Title |
Getting the Most
Bang for Your Buck: The Efficient Use of
Crowdsourced Labor for Data Annotation
Note: The
planned talk 'Crowdsourcing,
Human Computation, and Collective Intelligence'
will be held as a WORLDCOMP keynote on Monday,
July 16 in the morning. |
Date & Time |
Tuesday,
July 17, 04:40pm (approx. 60 min talk
and Q&A) |
Location |
Ballroom 1 |
Description |
The introduction
of crowdsourcing platforms such as Amazon
Mechanical Turk has made it possible to harness
cheap, albeit sometimes unreliable, human labor
in a range of data annotation tasks. The most
common approach for improving the quality of
annotations is to seek multiple annotations for
each item and use majority vote to assign a
final output. However, each annotation costs
money, and we want to make sure we spend that
money in the most effective ways possible. For
example, if you are given a fixed budget, what's
the best way to allocate data annotation work to
crowdsourced labor to achieve the most accurate
results that you can? This talk will discuss
recent results on how we can improve the labor
efficiency of crowdsourced data annotation tasks. |
Short Bio |
Haym Hirsh is
Professor of Computer Science at Rutgers
University. His research has focused on
foundations and applications of machine learning,
data mining, information retrieval, and
artificial intelligence, especially targeting
questions that integrally involve both people
and computing. Most recently these interests
have turned to crowdsourcing, human computation,
and collective intelligence. From 2006-2010 he
served as Director of the Division of
Information and Intelligent Systems at the
National Science Foundation, and from 2010-2011
he was a Visiting Scholar at MIT's Center for
Collective Intelligence. Haym received his BS
from the Mathematics and Computer Science
Departments at UCLA and his MS and PhD from the
Computer Science Department at Stanford
University. |
Invited Talk C |
Speaker |
Peter Geczy, AIST, Japan |
 |
Topic/Title |
Data Mining
in Organizations, Quo Vadis? |
Date & Time |
Wednesday,
July 18, 03:20pm (duration:
approx. 1 hour) |
Location |
Ballroom 1 |
Description |
Data mining has
been progressively expanding from academia and
research to commercial and governmental
organizational environments. Wide-ranging data
mining techniques and analytics are finding
applicability in organizational arena. Numerous
organizations have been considerably benefiting
from implementation of data mining solutions and
analytics. However, despite significant advances
in data mining techniques, and their efficient
implementation methods, majority of
organizations are still reluctant to adopt them.
What limits adoption of data mining techniques
in real-world organizational environments? Which
data mining techniques are currently at the
forefront of acceptance by diverse organizations?
What are the promising trends? These are the
pertinent questions we shall explore—after a
concise overview of the status quo. |
Short Bio |
Dr. Peter
Geczy is a chief scientist at The National
Institute of Advanced Industrial Science and
Technology (AIST). He also held positions at The
Institute of Physical and Chemical Research
(RIKEN) and The Research Center for Future
Technologies. His interdisciplinary scientific
interests encompass domains of data and web
mining, human interactions and behavior, social
intelligence technologies, privacy, information
systems, knowledge management and engineering,
artificial intelligence, and adaptable systems.
His recent research focus also extends to the
spheres of service science, engineering,
management, and computing. He received several
awards in recognition of his accomplishments.
Dr. Geczy has been serving on various
professional boards and committees, and has been
a distinguished speaker in academia and
industry. |
|
|